K. S. Ahmed
Department of Bio-electronics, Faculty of Engineering, MTI, Modern University for Technology and Information, Katamia, Egypt
Correspondence to: K. S. Ahmed , Department of Bio-electronics, Faculty of Engineering, MTI, Modern University for Technology and Information, Katamia, Egypt.
Email: |  |
Copyright © 2012 Scientific & Academic Publishing. All Rights Reserved.
Abstract
Many disorders can disrupt the neuromuscular channels used by the brain to communicate with and control its external environment. Patients with severe neural disorders lose most of voluntary muscle control. Some patients can control their eye movements and may be able to communicate. In the absence of methods for repairing the damage done by these disorders, the only option for restoring function to those with motor impairments is to provide the brain with a new, muscular/non-muscular and non invasive communication and control channel, a direct Brain Machine Interface (BMI) for conveying messages and commands to the external world. An attempt has been provided to collect the brain activities and extract specific estimations to control the wheelchair movement. The data has been collected though fourteen electrodes fixed on the scalp by modern strategy (without non-drying conductive past). This method has used a wireless communication head set based on the hydration sensors and Bluetooth technology. Four movements are detected (turn right – turn left – forward - stop) based on the eye blinks (right wing, left wing, single/double blinks). The wavelet has used to extract best signals and NN system to take the learning sessions and give the required action.
Keywords:
EEG, Wheelchair Movement, Wavelet, NN
Cite this paper:
K. S. Ahmed , "Wheelchair Movement Control VIA Human Eye Blinks", American Journal of Biomedical Engineering, Vol. 1 No. 1, 2011, pp. 55-58. doi: 10.5923/j.ajbe.20110101.09.
1. Introduction
People with severe motor disabilities and severe injuries (i.e., Spinal cord injuries, high cervical injuries, cerebral palsy, multiple sclerosis or muscular dystrophy) have a very hard movement. They face daily obstacles and challenges in living their lives as normal people. In these cases the communication systems based on brain activity play an important role and provide a new form of communication and control, either to increase the integration into the society or to provide to these people tools for interaction with their environment without a continued assistance. There are various techniques and paradigms in the implementation of brain-computer interfaces (BCI). A brain-computer interface is a communication system for generating a control signal from brain signals such as EEG and evoked related potentials. The communication between the two essential parts of BCI (brain and computer), is governed by the fact that the brain generates the command and the computer must interpret[1]. Although there are different methods dealing with brain, they are not practical to implement a human-machine interface. The reason is depending these methods on the anatomical information, other techniques are very invasive or have a lot of exposure to radiation and/or very expensive[2,3].So work with electroencephalographic (EEG) is the most convenient method and therefore the BCI is based on detecting the EEG signals associated with certain mental states. The paper is organized as: an introduction to EEG signals and activities is introduced. Later on an overview about the electrode positions and BCI technique is presented. Finally, the methodology and the collected results will be discussed.
1.1. The Electroencephalogram (EEG)
The electroencephalogram (EEG) is the study of brain function that reflects the brain's electrical activity. To collect brain electrical signal, electrodes placed on the scalp. The electrical signal is a scale of micro volts which can be recorded and analyzed. EEG signals have different rhythms described by variable ranges of frequency bands and voltages. Table-1 indicates the most common rhythms including the frequency range, the amplitudes, the prominence areas and the human conditions[1-7]. Table 1. EEG Rhythms with the common characteristics |
| Rhythm | Frequency range | Amplitude | Prominence area | Human status | Delta | 0.5-3hz | 20-400µV | variable | Asleep | Theta | 4-7hz | 5-100 µV | Frontal - Temporal | Awake-stress | Alpha | 8-13hz | 5-100 µV | Occipital- Parietal | Awake-Relax-closed eye | Beta | 14-30hz | 2-10 µV | Pre central-frontal | Awake –no movement | Gamma | >30hz | 2-10 µV | Pre central-frontal | A wake |
|
|
1.2. Electrode Positions
The 10-20 system or International 10-20 system is an internationally recognized method to describe and apply the location of scalp electrodes in the context of an EEG test or experiment. The "10" and "20" refer to the fact that the actual distances between adjacent electrodes are either 10% or 20% of the total front-back or right-left distance of the skull as shown in Figure.1. Each site has a letter to identify the lobe and a number to identify the hemisphere location. 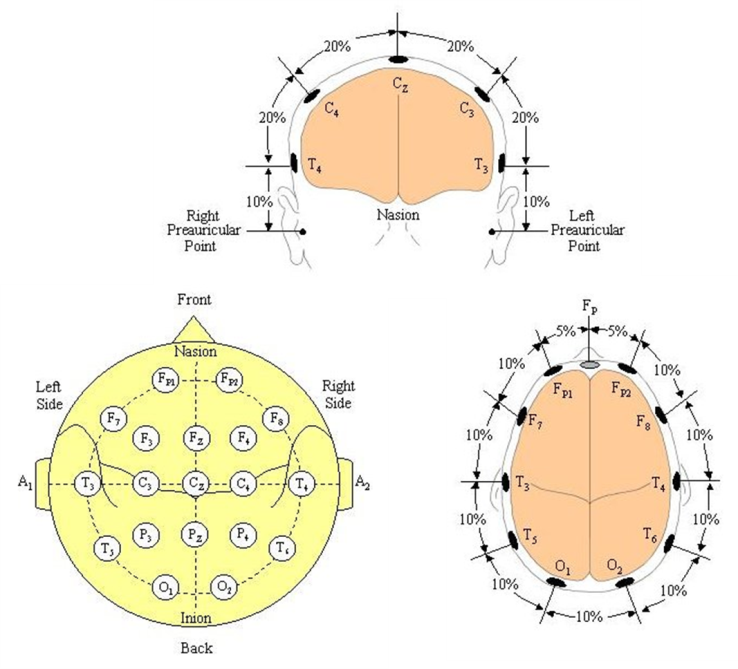 | Figure 1. a, the top view of electrode positions, b the front view and c for the contour percentage. |
1.3. Brain Computer Interface
A Brain Computer Interface (BCI) is an external device that communicates directly to the brain of humans or animals through neuron silicon interfaces. These external devices can either transmit or receive signals to and from the brain which can then be used to restore function or movement to sensory organs or limbs. Furthermore, these external devices can range from simple circuits to advanced silicon chips. As of today, brain computer interface devices have been successfully restoring damaged sight, movement and hearing. There are different types of BCI as Invasive Brain Computer Interfaces, Partially Invasive Brain Computer Interfaces and non Invasive Brain Computer Interfaces.
2. Methodology
The objective is to control the direction of an electric wheelchair using only EEG signals. In other words, this is an attempt to use brain signals to control mechanical devices such as wheelchairs. To achieve this goal, we have developed a recursive training algorithm to generate recognition patterns from EEG signals. Our experimental attempts demonstrate the utility of the proposed recursive training algorithm and the viability of accomplishing direction control of an electric wheelchair by only EEG signals. | Figure 2. The general block diagram of BCI. |
The paper introduces a prototype of a Brain Controlled Wheelchair (BCW) that can navigate inside a typical office and hospital environment with minimum structural modification. It is safe and relatively low cost and provides optimal interaction between the user and wheelchair within the constraints of brain computer interface. In this study, Wavelet Transform (WT) method was used for feature extraction of mental tasks from eight channel EEG signals. WT coefficients give the best discrimination between the directions of wheelchair in the relevant frequency band. The WT coefficients were used as the best fitting input vector for classifier. Radial Basis Function network was used to classify the signals. On the BCI side, a new technology is available every year, so the method depends on a wireless head set used to record the brain activity as shown in Figure.3. Such devices do not require conductive gel, hence should be easier to use and more comfortable. Besides they should be smaller and cheaper than current devices. However, the quality of the measurement will have to be assessed first.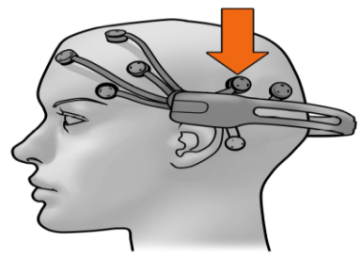 | Figure 3. The left hand side of the used head set |
Data collected from right-handed healthy male subjects of age (mean: 23yr) having no sign of any motor- neuron diseases were selected for the study. The participants were student volunteers for their availability and interest in the study. Data from subjects performing one task were analyzed. The following task were used to record the appropriate EEG data and each session takes 20 seconds with 4 actions each action takes 5 seconds as shown in the following table Table 2. The required action and the related action |
| Action | Function/Action | Left wink | move left | Right wink | move right | Blink | move forward | Double blink | move backward |
|
|
The data collected from two electrodes F7 and F8 as shown in Figures.3 and 4. That is because they give the best response of eye movement.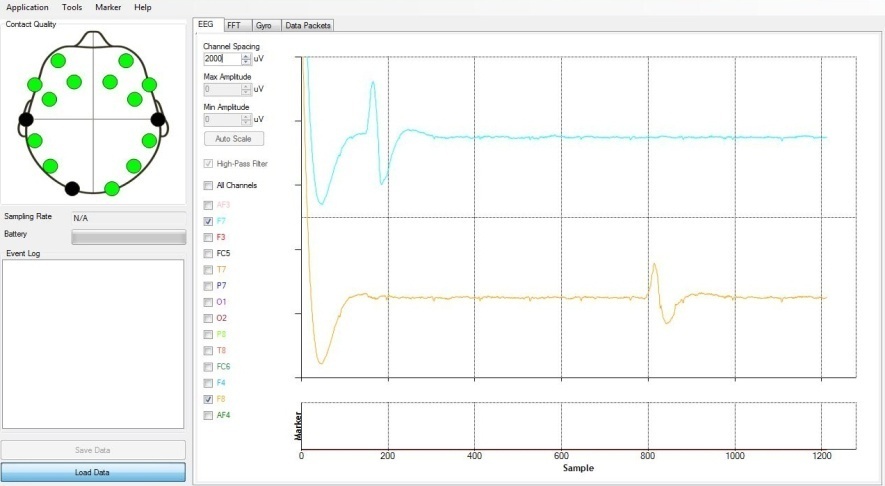 | Figure 3. The first part of blue signal is related to right wink and the red signal response for the left wink. |
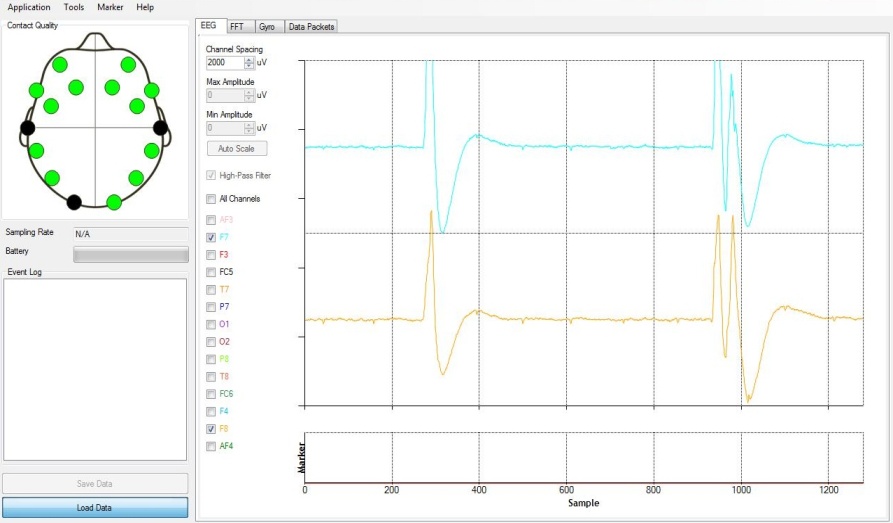 | Figure 4. The first part of blue and red signals is related to one blink and the second response for the double blinks. |
2.1. Feature Extraction
The wavelet transform (WT) was designed specifically to work with signals that have non-stationary properties, and, due to the characteristics of EEG, it is a very suitable tool for feature extraction in BCI designs[8-13]. The DWT was successfully used to extract information from EEG signals using symmetric electrode pairs. The weighted energy difference between electrodes pairs were used as features. Signals are recursively decomposed into high and low passed sub-bands, and the resolution of the spectrum is determined by the chosen decomposition level. The sub-band energy from the last decomposition level was used to construct features from EEG signals. Two feature types were used for this study. The first involved signal is the band power, and was calculated with wavelet decomposition. The second type was constructed from the spectral coefficients in the buffer. The methods were all causal and could be used in a real-time environment.
2.2. Classification
Classification of signal segments into a given number of classes using segments features can be achieved by various statistical methods. In our study, the classification is based upon the application of self-organizing neural networks using features as patterns for the input layer of neural network. The number of output layer elements is equal to signal classes and must be either defined in advance or it can be automatically increased to create new classes. The technique is found a linear hyper plane Hs to separate the vectors from different classes in their feature space.
3. Results
The study introduced a prototype for wheelchair movement control via eye blinks through EEG electrodes. The signals were collected from healthy volunteers and were analyzed through wavelet technique. The classification is performed through NN system to determine the required motion after the learning process. The output was determined via groups for every action and indicating the related event. The sensitivity and specificity were calculated for 20 cases and there were 80% and 75% respectively.
4. Discussion
This paper introduced a prototype for wheelchair movement control via eye blinks through EEG electrodes. Although the system dependent on the strength of the electrical activities, eye blinks and winks which may be not suitable for some patients, and the sensitivity and specificity are roughly low, the system is considered as a seed to establish completed learning system for the disorder patients. The system will take all the nerve irritations and to be feedback and real time system.
References
[1] | Basic Anatomy and Physiology of the Human Brain http://medphys.ucl.ac.uk/research/borg/homepages/............/p25_34.pdf |
[2] | E. R. Kandel, J. H. Schwartz, and T. M. Jessell, “Principles of Neural Science”, 1991 |
[3] | W. W. Orrison Jr., J. D. Lewine, J. A. Sanders, and M. F. Hartshorne, “Functions Brain Imaging”, 1995 |
[4] | F. H. Lopes da Silva and A. van Rotterdam. “Biophysical aspects of EEG and MEG Generation”, 1982 |
[5] | The "10-20 System" of Electrode Placement. http://faculty.washington.edu/chudler/1020.html |
[6] | J. R. Wolpaw, D. J. McFarland, G. W. Neat, and C. A. Forneris. "An EEG-based brain computer interface for cursor control", 1991 |
[7] | G. Pfurtscheller, J. Kalcher, Ch. Neuper, D. Flotzinger, and M. Pregenzer, "On-line EEG classification during externally-paced hand movements using a neural network based Classifier”, 1996. |
[8] | N. Birbaumer, N. Ghanayim, T. Hinterberger, I. Iversen, B. Kotchoubey, A. Kubler, J. Perelmouter, E. Taub, H. Flor. "A Spelling Device for the Paralysed", 1999 |
[9] | S. Lin, Y. Tsai, and C Liou, "Conscious mental tasks and their EEG signals", 1993 |
[10] | Gerwin Schalk, Dennis J. McFarland, and Jonathan R. Wolpaw, “Brain computer interface for communication and control”, 2006 |
[11] | E. E. Sutter, “The brain response interface: communication through visually-induced electrical brain responses", 1992 |
[12] | K. S. Jones, M. S. Middendorf, G. Calhoun, and G. McMillan, "Evaluation of an Electroencephalographic-based Control Device", 1998 |
[13] | M. S. Middendorf, G. McMillan, G. Calhoun, and K. S. Jones, “Brain-computer interfaces based on the steady-state visual-evoked response”, 1999 |