Vijaykumar H. K.
Department of Mechanical Engineering, Yenepoya Institute of Technology, Moodbidri, Mangaluru, India
Correspondence to: Vijaykumar H. K., Department of Mechanical Engineering, Yenepoya Institute of Technology, Moodbidri, Mangaluru, India.
Email: |  |
Copyright © 2016 Scientific & Academic Publishing. All Rights Reserved.
This work is licensed under the Creative Commons Attribution International License (CC BY).
http://creativecommons.org/licenses/by/4.0/

Abstract
The use of Polytetrafluoroethylene (PTFE) tubes has broadened across various applications including automotive, chemical, electrical and medical. PTFE tubes are mainly manufactured by extrusion process. Shore hardness of PTFE tube is a mechanical property to be mainly considered. Shore hardness of the tubes depends on temperature of the extrusion and sintering temperature. In this paper, prediction of shore hardness of PTFE tubes is carried out using Artificial Neural Network (ANN). Use of ANN in prediction provided the results which are close to the actual results. The results conclude that the ANN is superlative tool which suits to envisage the shore hardness of PTFE tubes.
Keywords:
Temperature, Sintering, Prediction
Cite this paper: Vijaykumar H. K., Employing the Artificial Neural Network to Envisage the Shore Hardness of Polytetrafluoroethylene Tubes by Extrusion, Journal of Mechanical Engineering and Automation, Vol. 6 No. 5A, 2016, pp. 54-57. doi: 10.5923/c.jmea.201601.10.
1. Introduction
Polytetrafluoroethylene (PTFE) a fluorocarbon-based polymer is a resin supplied in powdered form, which is mixed, preformed, and extruded into a paste, and then finally tubing [1]. PTFE tubing can be used in a large variety of applications due to its high chemical resistance, high and low temperature capability, resistance to weathering, electrical and thermal insulation, and lowest coefficient of friction of any solid material. The tubing is translucent white in colour [2]. The use of PTFE tubing has spread across various applications including automotive, chemical, electrical and medical. In automotive applications, the ability of PTFE to withstand temperatures in excess of 250°C makes it an ideal candidate for high temperature fluid transfer. In medical applications, PTFE tubing is in huge demand due to its lubricant and chemical inertness [3-7]. Catheters employing PTFE tubing can be inserted into the human body without fear of reaction or abrasion with any body parts. In chemical applications including laboratories PTFE is an ideal replacement for glass due to its inertness and durability [8]. In electrical applications, the excellent dielectric properties of virgin PTFE make it well suited for insulating high voltage cables [9-12]. Figure 1 shows the image of the extruded Polytetrafluroethylene tube.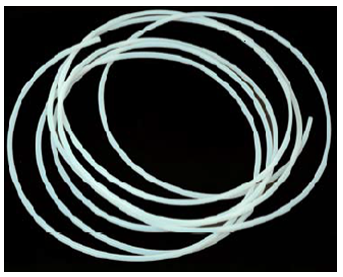 | Figure 1. Image of Extruded PTFE tube |
2. Factors Affecting Shore Hardness
The factor which mainly affects the shore hardness of PTFE tube produced by extrusion mainly includes the extrusion temperature and sintering temperature [1]. The shore hardness data obtained based on extrusion and the sintering temperature are as shown in the table 1. The extrusion temperature and sintering temperature is recorded and the corresponding hardness obtained for the tube is measured in D scale of Durometer. Twenty one observations were taken for the study.Table 1. Shore hardness Data obtained based on extrusion and sintering temperature 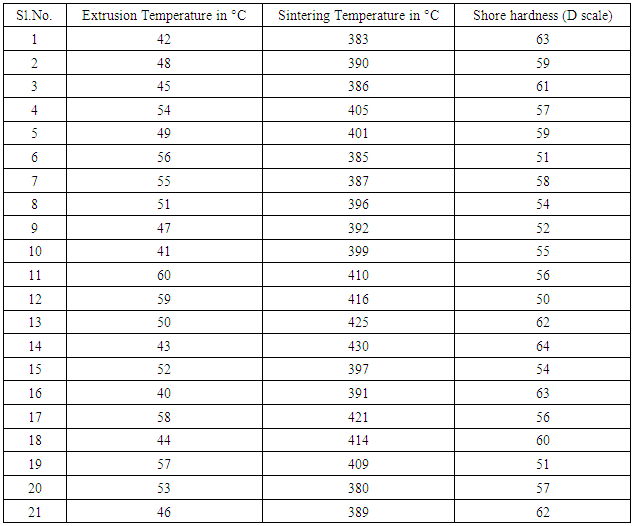 |
| |
|
3. Employing ANN for Prediction of the Shore Hardness
The data obtained from observations is used for training and developing the ANN model using Matlab. The 75% of data is used for training the ANN model and remaining 25% is used for validation of the developed ANN model. The modus operandi includes the following steps: 1) Choosing the training and testing data: The 75% of observation is used for training the ANN. The table 2 shows the training data and remaining 25% is used for testing. The 75% of training data i.e. 15 observerations were used for training the neural network and 6 observations were used for the testing the neural network developed using Matlab software.Table 2. Training data for ANN model 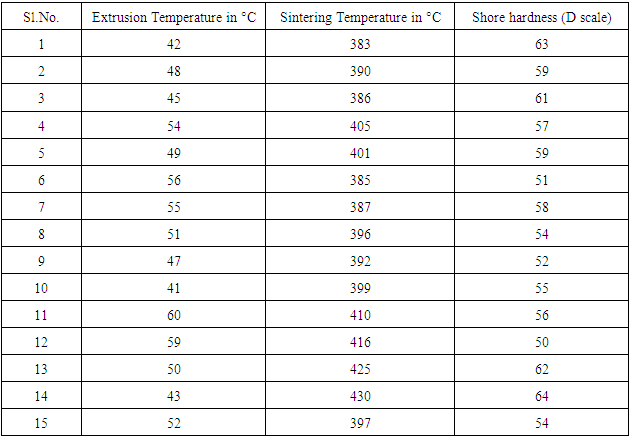 |
| |
|
2) Normalization of training data: Normalization is done by dividing the maximum value. Table 3 shows the normalization of the data.Table 3. Normalized data for ANN model 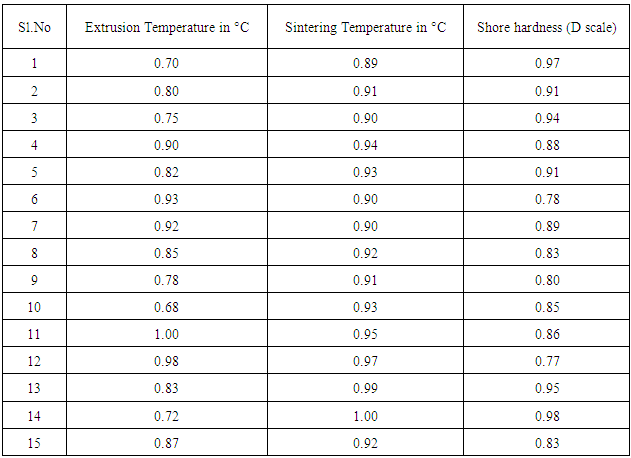 |
| |
|
3) Development of ANN model: The artificial neural network used in modelling of shore hardness contains three layers, (two input neurons, two layers of seven hidden neurons and one output neuron). Figure 2 shows network architecture for shore hardness. This is developed in the Matlab the feed forward network back-propagation ANN model is developed and trained using normalized investigational data. Normalization is a vital step in model development to give almost equal weight age to all the variables. To normalize the different input variable following maximum limits are taken respectively in same. The development step includes the Selection of suitable ANN structure, Training and development of model, Test run of model, Simulation and results.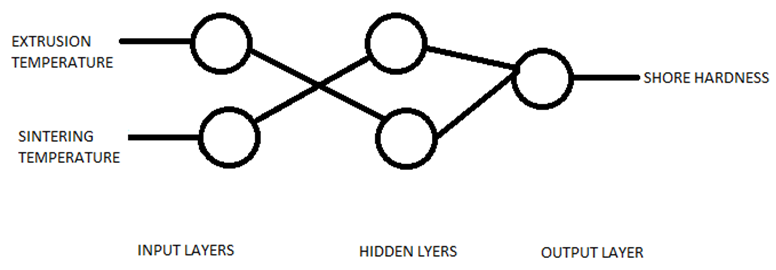 | Figure 2. Network Architecture for Shore Hardness |
4. Results
The results obtained after development of ANN model is tested with remaining data on testing the ANN model provided the following result with an error of 6.66% which is acceptable range of ±10%. The table 4 shows actual the shore hardness and predicted shore hardness.Table 4. Prediction data of ANN model 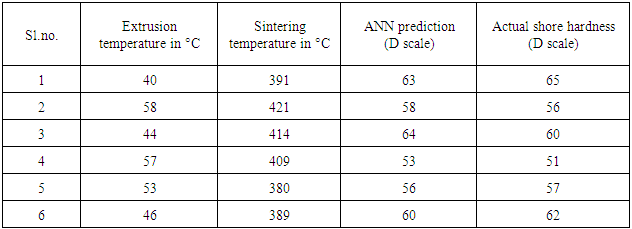 |
| |
|
The table 5 shows the percentage of error occurred from ANN model developed. Table 5. Error in terms of Percentage from ANN  |
| |
|
5. Conclusions
The ANN model developed for predicting of shore hardness is well within the range of ±10% which is acceptable from the results obtained therefore the developed model can be used for predicting the shore hardness of PTFE tubes. It also indicates the Extrusion and sintering temperature are the two important factors for shore hardness of the PTFE tubes manufactured from the Extrusion process. Finally it can be said that ANN is a superlative tool can be used for prediction of mechanical property like shore hardness in manufacturing industries.
ACKNOWLEDGEMENTS
The author is grateful to Polyfluoro India Ltd., Bengaluru for providing facilities to carry out this work. Author is also thankful to Yenepoya Institute of Technology Moodbidri, Mangaluru for encouragement and support.
References
[1] | E. W. Rowne, “Shore hardness studies of PTFE materials”, International Journal of Polymers, vol.6, pp79-86, 1999. |
[2] | Y. Kernad and T. Venagy. “Some studies on mechanical properties of Teflon”, Russian Engineering Research, vol. 12, No. 4, pp. 32–37, 2000. |
[3] | Patrick K. Simpson, “Artificial Neural Systems”, Pergamum Press, NewYork, pp. 112-123, 1990. |
[4] | D. S. Levine, “Introduction to Neural & Cognitive Modelling”, Lawrence Erlbaum Associates, Publishers, Hillsdale, pp. 58-62, 2000. |
[5] | Stephen I. Gallant, Chapters 11 & 12; “Neural Network Learning and Expert Systems”, The MIT Press, Cambridge, MA, 1993. |
[6] | R.K. Goyal, and M. Yadav, ‘‘Study on wear and friction behaviour of graphite flake-filled PTFE composites’’, Journal of Applied Polymer Science, Early View, May 2012. |
[7] | Y.R. Kharde and K.V. Saisrinadh “Effect of oil and oil with graphite on tribological properties of glass filled PTFE polymer composites”, Indian Academy of Sciences, vol. 34, No. 4, pp. 1003–1012, July 2011. |
[8] | LIN Rui, ZHAO Tiantian, ZHANG Haiyan, CAO Chunhui1, LI Bing and MA Jianxin “Influence of PTFE on Electrode Structure for Performance of PEMFC and 10-Cells Stack”, Chinese Journal of Mechanical Engineering, vol. 25, No. 6, pp1171-1175, 2012. |
[9] | Conor F. Dunne, Kevin Roche, Barry Twomey, Darel Hodgson and Kenneth T. “Blast Coating of Superelastic NiTi Wire with PTFE to Enhance Wear Properties”, ASM International Journal, pp41-49, 2015. |
[10] | D. A. Negrov and E. N. Eremin, “Manufacture of Slip Bearings from PTFE Based Composite”, Russian Engineering Research, vol. 32, No. 1, pp. 42–44, 2012. |
[11] | S. M. Patil and B. B. Ahuja, “Tribological Behaviour of PTFE under Variable Loading Dry Sliding Condition”, The Institution of Engineers (India), pp179-185, Apr 2014. |
[12] | Mitsuhiro Horade and Susumu Sugiyama, “Fabrication of 3-D PTFE microstructures utilizing change of etching rate with respect to exposure time”, Springer-Verlag, pp351–356, Jul 2012. |