Lea Tien Tay1, Mutasem Sh. Alkhasawneh1, Habibah Lateh2, Md Kamrul Hossain2, Anton Abdulbasah Kamil2
1School of Electrical and Electronic Engineering, USM Engineering Campus, Penang, Malaysia
2School of Distance Education, Universiti Sains Malaysia, Penang, Malaysia
Correspondence to: Lea Tien Tay, School of Electrical and Electronic Engineering, USM Engineering Campus, Penang, Malaysia.
Email: |  |
Copyright © 2014 Scientific & Academic Publishing. All Rights Reserved.
Abstract
Landslides in Malaysia are mainly triggered by rainfall. However, there are many other landslide causative factors which are related to landslide event in Malaysia. One of them is topographical factor which plays an important role in the landslide susceptibility analysis. This paper presents landslide hazard mapping using dominant factors with Poisson Distribution approach and the study area is Penang Island of Malaysia. Landslide hazard map of Penang Island is generated by taking into account of twenty-two landslide causative factors, including fourteen topographic factors. After considering all twenty-two factors for landslide hazard mapping, the analysis is repeated with removing one factor at one time to determine the dominant landslide-causative factors. Eleven dominant factors are selected from the twenty-two factors. Landslide hazard map was segregated into four categories of risks, i.e. Highly hazardous area, Hazardous area, Moderately hazardous area and Not hazardous area. The maps was assessed using ROC (Receiver Operating Characteristic) based on the area under the curve method (AUC). Landslide hazard map produced by including all 22 factors has an accuracy of 77.75%. By removing 11 irrelevant factors and employing only 11 dominant factors, the generated hazard map achieves better performance with accuracy of 79.20%.
Keywords:
Poisson Distribution, Geohazard, Hazard Mapping, Landslide
Cite this paper: Lea Tien Tay, Mutasem Sh. Alkhasawneh, Habibah Lateh, Md Kamrul Hossain, Anton Abdulbasah Kamil, Landslide Hazard Mapping of Penang Island Using Poisson Distribution with Dominant Factors, Journal of Civil Engineering Research, Vol. 4 No. 3A, 2014, pp. 72-77. doi: 10.5923/c.jce.201402.12.
1. Introduction
Landslide is a geological phenomenon involving movement of mass of rock, deep failure of slopes or debris flows due to soil erosion. Landslides cause infrastructure damages, destroy properties and even claim human lives. Landslides happen rather frequently in Malaysia due to the heavy rainfall especially during annual monsoons mainly known as Southwest Monsoon from late May to September, and Northeast Monsoon from November to March. Damages due to landslides have been high from 2000 to 2009 [1]. It is difficult to predict a landslide event in space and time, however an area may be classified into different categories based on the level of potential hazard due to mass movement [2]. The identification of high risk areas is important in landslide prediction and warning system. Penang Island is chosen as area of interest in landslide hazard analysis in this research work as it has suffered numerous damages due to landslide in recent years. There have been many studies on landslide hazard evaluation using Geography Information Systems (GIS) and various analysis techniques. Probabilistic methods have been applied to achieve promising result in landslide hazard analysis [1, 3-6]. In this study, Poisson distribution was applied to produce the landslide hazard map for Penang Island. To the best of our knowledge, the Poisson distribution has been used for landslide hazard mapping for the first time in our previous study [7].
2. Study Area
The study area chosen in this research work is Penang Island (Fig. 1) due to its frequent landslide occurrences over the years. Penang is one of the 13 states of Malaysia and it is located on the North West of Peninsular Malaysia. It is bounded to the north and east of the state of Kedah, to the south of the state of Perak and to the west of Strait of Malacca. Penang consists of the island of Penang and a coastal strip on the mainland known as Province Wellesley. In this paper, the island of Penang is considered for landslide analysis and mapping. The island covers an area of 285 km2 and is separated from the mainland by a channel. It is located on the geographical coordinates of 5o15’N to 5o 30’N latitudes and 100o 10’E to 100o 20’E longitudes. Elevation of the terrain ranges from 0 to 820 meters above sea level and slope gradient ranges from 0o to 87o. Penang Island is affected by fault lines that run from north to south mainly in the centre of the island. Land-cover of Penang Island consists mainly of swamp, plantation, forest, grassland and urban area. Major vegetation types are forest and fruit plantation. The average amount of rainfall varies from 2254 to 2903 mm annually. 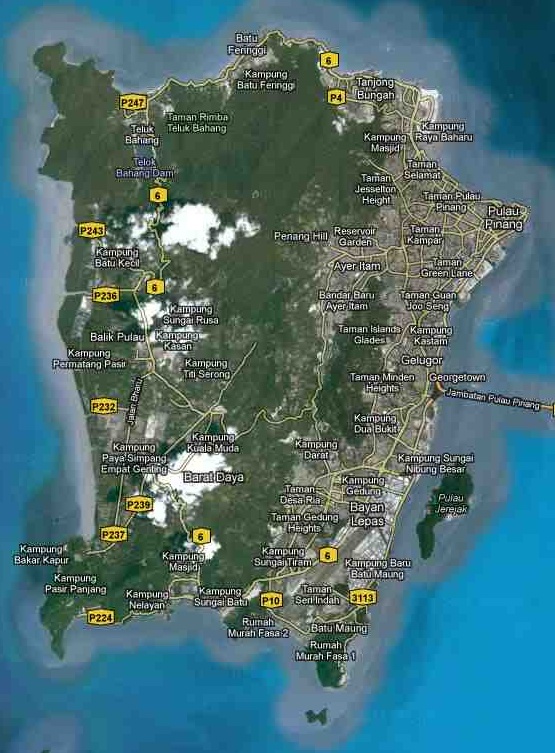 | Figure 1. Penang Island (Google map) |
Data collection of Penang Island database was carried out. Topographical, geological and various images of Penang Island were obtained from various government departments in Malaysia including Malaysia Meteorological Department (MMD), Department of Irrigation and Drainage (DID), Department of Agriculture (DOA), Minerals and Geoscience Department (JMG), Department of Survey and Mapping Malaysia (JUPEM) and Penang Geography Information System Center (PeGIS). Landslide occurrence points in Penang Island were also collected and transformed into spatial database for probabilistic landslide susceptibility analysis. Most landslides in Penang Island happened at the mountainous terrain which locates in the center of the island. These landslides consist of mainly shallow rotational debris slides and debris flows. Identification and mapping of a suitable set of landslide-causative facts having relationship with the slope failures requires prior information of the main causes of landslide [8]. In order to apply the probabilistic methods, data sets of landslide-causative factors were collected and developed as spatial database. In this research work, twenty two landslide-causative factors were considered in the computation of the landslides probability. From topographic database, digital elevation model (DEM) was constructed with a resolution of 10-meter. DEM provides the elevation of the study area. Elevation was used to calculate the slope angle, slope aspect, plan curvature, profile curvature, tangential curvature, longitudinal curvature, cross section curvature, general curvature, total curvature, diagonal length, surface area, surface roughness and rugosity [9]. Distance from drainage and distance from road were computed from drainage map and road map each available in digital map respectively. Van Westen et al. [10] suggested that buffer zones for line features, such as, rivers and roads should be set to 50 m. Similarly, distances from fault lines of Penang Island were calculated and divided into 100 m intervals. Soil texture database of the island includes sand, clay and urban land. Land use map consists of 17 classes of land cover and vegetation cover map consists of 14 classes of vegetation types. Geology map, tabling the types of rocks and granites of Penang Island is used as one of the landslide-causative factors. Rainfall or precipitation is the triggering factors of landslide as it saturates soils and slops away debris and rocks, causing landslides to happen. There are only several rain gauge stations in Penang Island. Therefore, interpolation method was used to prepare the precipitation map. Using 29 years of historical rainfall data (1980-2008), statistical distribution of the accumulated average precipitation was prepared using inverse weight distance interpolation method.
3. Methodology
Thirteen topographical factors were extracted from DEM, i.e. slope angle, slope aspect, plan curvature, profile curvature, tangential curvature, longitudinal curvature, cross section curvature, general curvature, total curvature, diagonal length, surface area, surface roughness and rugosity. Detailed works on the factors extraction can be found in [9]. Besides topographical factors, there are eight landslide-causative factors considered in this research work as well. They are land cover, vegetation cover, distance from road, distance from stream, distance from fault line, geology, soil texture and rainfall precipitation.Landslide hazard evaluation using probabilistic method has been proven to be very useful in the landslide prediction. The advantages of this method include its high efficiency, low cost, easy implementation and better interpretation of the relationships between landslides and landslide-causative factors. In probabilistic method, the weightages of each factor are calculated based on landslide density for certain classes [11]. These weightages represent the importance of each factor to occurrence of landslide and are used to produce landslide hazard index. Poisson distribution is a discrete distribution [12]. It is very useful in ecological studies. In this study, Poisson distribution is used for “landslide” which is a geological event. The distribution function for the Poisson distribution is defined as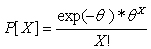 | (1) |
To estimate probability of one landslide under a characteristic, Poisson distribution is simplified as follows:  | (2) |
where 
Higher value of the probability for a characteristic shows high risk of landslide occurrence. Landslide hazard index is computed by summing up the probability of landslide in term of Poisson distribution of various factors as follows: | (3) |
Where P = Poisson probability value of each factor.First of all, all the twenty-two factors were considered in the landslide hazard analysis using the Poisson distribution. The landslide hazard map was generated and the assessment was carried out using ROC (Receiver Operating Characteristic) to check the accuracy of this output map. Secondly, the analyses were repeated by removing one factor at one time. The results were assessed with ROC to determine the dominant landslide-causative factors. If the accuracy declines when one particular factor is removed, that particular factor is a dominant factor in landslide hazard analysis. On the other hand, if the accuracy increases when a factor is removed, that factor is irrelevant. The irrelevant factors should be removed. The importance of each factors is ranked based on the accuracies obtained. Finally, the selected dominant factors are used in the probabilistic method for landslide hazard analysis to produce the final landslide hazard map.
4. Verification and Discussion
In our analysis, ROC method was employed to verify the efficiency and accuracy of the landslide hazard mapping. Two assumptions are necessary to verify the maps. First assumption is that landslides are related to spatial information such as slope gradient and slope aspect, and the second assumption is that the future landslides will be affected by triggering factor such as rainfall [13]. Both assumptions are fulfilled in this study. Verification by ROC is carried out by first sorting the landslide hazard indexes (LHI) in a descending order. The ordered indexes are then divided into 100 classes and set on y-axis, with accumulated 1% intervals on x-axis [1]. The resulting graph shows a line curve that explains how well the model and factors predict future landslide [13]. The accuracies of the prediction models is represented by the area under the curves and it is one of the commonly used accuracy statistics in natural hazard assessments [13]. Table 1 shows the results obtained from the assessment of the landslide hazard map produced using all twenty-two factors and also the maps produced by removing one factors at one time. The accuracies of the landslide hazard maps are presented in percentage. If the accuracy of the produced map decreases when one particular factor is removed, this particular factor is a dominant landslide-causative factor. One the other hand, if the accuracy of the produced map increases by removing a particular factor, that factor is unimportant in landslide hazard analysis. Therefore, those unimportant factors should be removed to produce a hazard map with better accuracy. Based on the results in Table 1, eleven dominant factors are selected and they are distance from distance from drainage, rugosity, height, slope gradient, diagonal length, surface area, distance from fault line, rain, aspect, geology and soil texture. The unimportant factors are plan curvature, tangential curvature, cross section curvature, vegetation, total curvature, distance from road, general curvature, profile curvature, landuse, longitudinal curvature and surface roughness. The factors are arranged in the level of their importance in landslide analysis in Table 1, from the most important factor (distance to drainage) to the two least important factors (tangential curvature and plan curvature).Table 1. Accuracies of landslide hazard map produced by using all factors and also removing one factor at one time 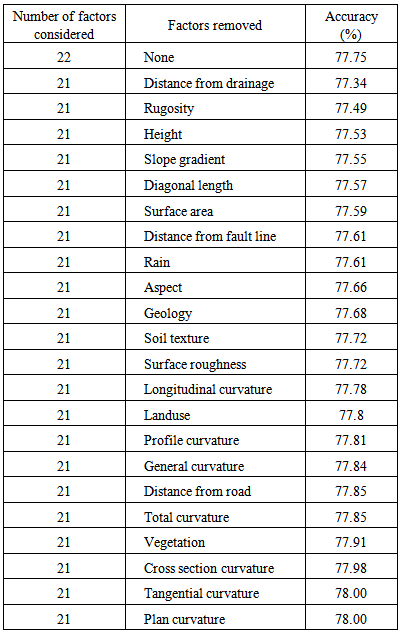 |
| |
|
Figure 2 shows the accuracies of landslide hazard maps produced with lesser number of factors from 22 to 6. Firstly, the most unimportant factor is removed. It is followed by removing two unimportant factors until 16 factors are removed based on the sequences of their irrelevancy in Table 1. The accuracies increase by removing more unimportant factors. However, the accuracies reduces when important factors are removed too. Landslide hazard map produced using Poisson distribution method achieves an accuracy of 77.75% when all twenty-two factors are considered. When 11 unimportant factors were removed, the final hazard map has best accuracy of 79.20% using Poisson distribution with the eleven dominant factors.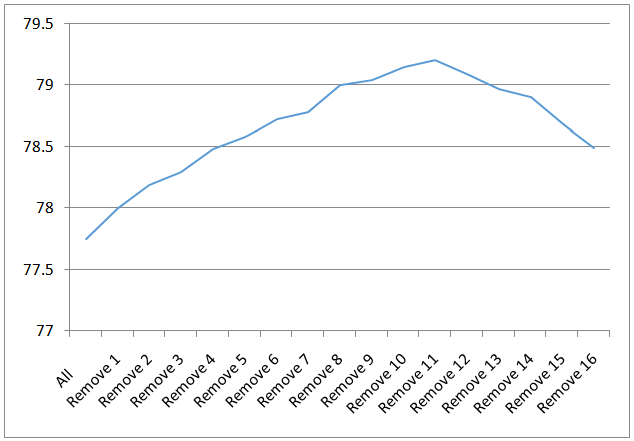 | Figure 2. Accuracies of the landslide hazard maps produced by removing increasing number of factors |
Landslide hazard mapping is done by classifying LHI (landslide hazard index) into several categories of risks. In this study, the indexes are classified into 4 groups: Highly hazardous for the highest 10% of the indexes (90-100%), Hazardous for the next 10% (80-90%), Moderately hazardous for the next 20% (60-80%) and Not hazardous for the remaining 60% (0-60%). ROC curves illustrate how well the prediction models fit the data of landslide-causative factors with landslide occurrences to predict future landslides. Figure 3 shows the landslide hazard maps produced using Poisson distribution with all twenty-two factors and with eleven dominant factors. Red color represents “Highly hazardous” areas, green color indicates “Hazardous” areas, blue color represents “Moderately hazardous” areas and “Not hazardous” areas are represented by white color.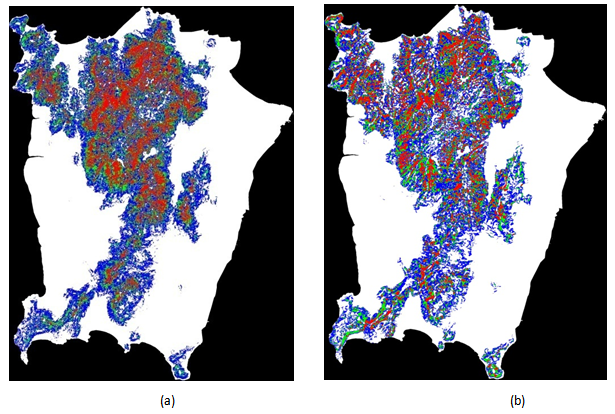 | Figure 3. Landslide hazard maps produced using Poisson distribution (a) with all twenty-two factors, (b) with eleven dominant factors |
5. Conclusions
There are a lot of housing and development in the mountainous areas of Penang Island due to the limitation of flat land. This creates a scenario where landslide warning system is required to warn people of impending landslides on hazardous area. Prediction models such as statistical or probabilistic methods are simple approaches which have been proven to produce promising results in landslide prediction. In this paper, landslide hazard maps of Penang Island were produced with Poisson distribution using twenty-two landslide-causative factors available and also eleven dominant factors. The accuracy of the produced landslide hazard map improves when eleven dominant factors are used in the landslide hazard analysis as compared to the map produced using all twenty-two factors. The produced maps are useful as part of the guidelines in the sustainable land use planning and future development.
ACKNOWLEDGMENTS
The authors would like to thanks the sponsorship from Ministry of Education Malaysia (MOE) under FRGS Top-Down research grant. The authors would also like to thank the Minerals and Geosciences Department Malaysia and Department of Irrigation and Drainage Malaysia for the data used in this research.
References
[1] | B. Pradhan and S. Lee, “Delineation of landslide hazard areas on Penang Island, Malaysia, by using frequency ratio, logistic regression, and artificial neural network models,” in Environmental Earth Sciences, Volume 60, Number 5, 1037-1054, 2010. |
[2] | D. J. Varness, “Landslide hazard zonation: a review of principles and practice,” in Nat Hazards, 3:63, 1984. |
[3] | S. Lee and J. A. Talib, “Probabilistic landslide susceptibility and factor effect analysis,” in Environ. Geol., 47, pp 982-990, 2005. |
[4] | A. K. Saha, P. R. Gupta, I. Sarkar, M. K. Arora and E. Csaplovics, “An approach for GIS-based statistical landslide susceptibility zoning-with a case study in the Himalayas” in Landslide, 2(1), pp. 61-69, 2005. |
[5] | K. W. Lim, L. T. Tay, H. Lateh, “Landslide Susceptibility Mapping of Penang Island using Probabilistic Method and Logistic Regression”, IEEE International Conference on Imaging Systems and Techniques (IST 2011), 17-18 May 2011. |
[6] | Lea Tien Tay, Habibah Lateh, "Landslide Hazard Mapping Using Probabilistic Approaches: A Case Study of Penang Island, Malaysia", Caspian Journal of Applied Sciences Research, 2 (AICCE'12 & GIZ'12), pp. 140-144, 2013. |
[7] | Lea Tien Tay, Habibah Lateh, Md Kamrul Hossain, Anton Abdulbasah Kamil, “Landslide Hazard Mapping Using a Poisson Distribution: A Case Study in Penang Island, Malaysia”, Landslide Science for a Safer Geoenvironment, Springer International Publishing, pp. 521-525. |
[8] | F. Guzzetti, A. Carrarra, M. Cardinali, P. Reichenbach, “Landslide hazard evaluation: a review of current techniques and their application in a multi-scale study, Central Italy,” in Geomorphology, 31, pp 181-216, 1999. |
[9] | Mutasem Sh. Alkhasawneh, Umi Kalthum Ngah, Lea Tien Tay, Nor Ashidi Mat Isa, “Determination of importance for comprehensive topographic factors on landslide hazard mapping using artificial neural network”, Environmental Earth Sciences, DOI 10.1007/s12665-013-3003-x, 2013. |
[10] | C. J. Van Westen, N. Rengers, R. Soeters, “Use of geomorphological information in indirect landslide landslide susceptibility assessment,” in Natural Hazards, 30, pp 399-419, 2003. |
[11] | M. L. Suzen and V. Doyuran, “A comparison of the GIS based landslide susceptibility assessment methods: multivariate versus bivariate,” in Environmental Geology, 45, pp 665-679, 2004. |
[12] | Frank A. Haight, Handbook of the Poisson Distribution. New York: John Wiley & Sons. |
[13] | C. F. Chung, A. G. Fabbri, “Probabilistic prediction models for landslide hazard mapping,” in Photogrammetric Eng Remote Sens, 65(12), pp 1389-1399, 1999. |